For a few years now, the team from fast.ai has been providing free education about deep learning on their website. Their video course promises a hands-on approach that aims to de-mystify the technologies of modern deep learning. With the book “Deep Learning for coders with fast.ai“, they bring these education principles to the written format, either as a printed book from O’Reilly or on Github (for free).
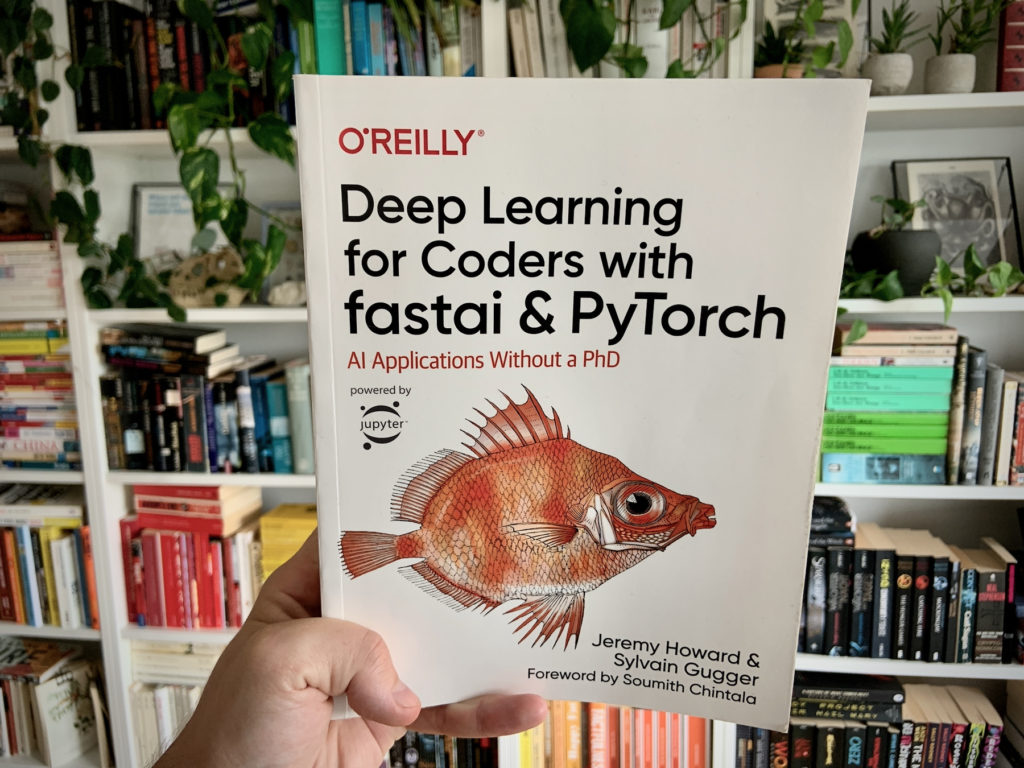
Before I talk about the book, some context: fast.ai is the name of a website with a video course of the same name. The course is taught using a Python library (called fastai, no dot) which is built on top of PyTorch, the popular deep learning framework. Nomenclature can be confusing. I’ll try to be specific and reference “the fast.ai team” or “the fastai library” in this review of the book.
Teaching structure: Top-down, then bottom-up
The authors are very vocal about their teaching principles: The goal is to “teach the whole game” while skipping the often demotivating mathematical principles at the beginning.
Instead, the first example gives you all instructions needed to train a state-of-the-art image classification model from scratch.
Then, the book progresses deeper into the technical and mathematical foundations, which they use to build up a (simple) version of their fastai library from scratch.
I’m torn: While this structure lowers the barrier of entry, it also makes for a repetitive experience: You encounter the same example many times, just at different levels of abstraction.
What’s in the book
The book covers a wide range of deep learning topics at different levels of depth.
You see practical examples across the main applications areas of deep learning: Computer vision, natural language processing, tabular modeling, and collaborative filtering
All examples are presented with full code listings and everything in the book invites you to go and try things out yourself.
The book presents a wide collection of deep learning techniques that help to get trainings running properly in practice.
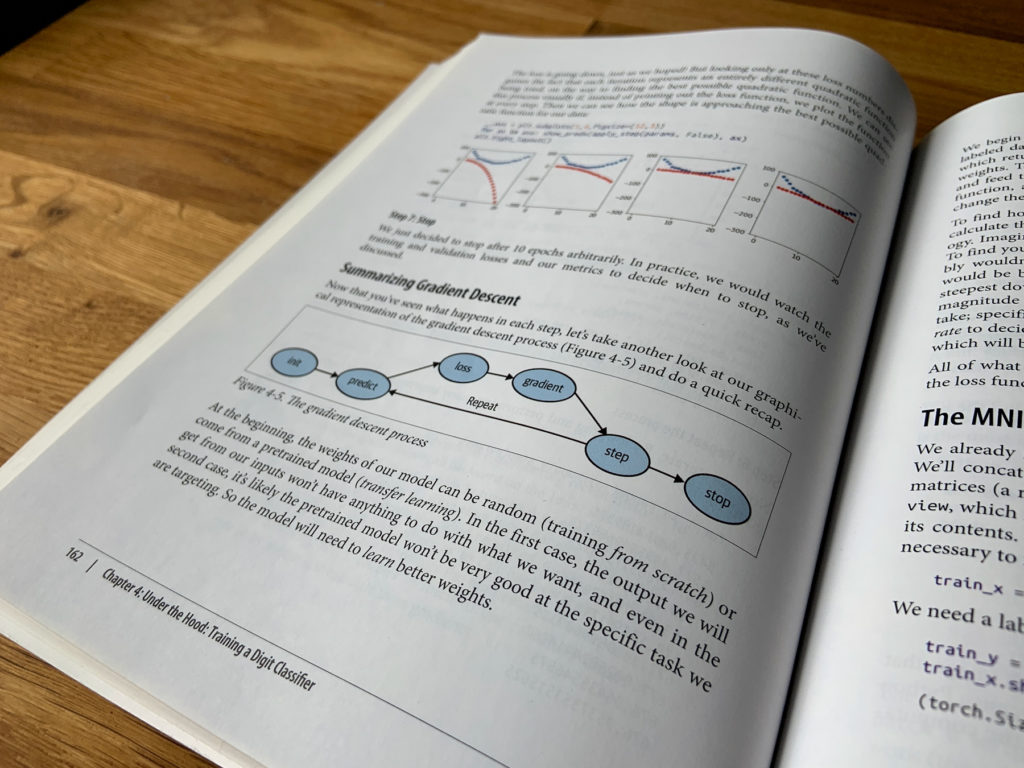
Popular deep learning architectures are explained, including ResNet, LSTMs, and U-Nets. With a mix of code, visualization, and (some) maths, the authors do a good job of conveying the core ideas of important architectures.
The authors don’t stop at the technical explanations but stress that it’s important to think further. Deep learning is a powerful tool and one that should be used responsibly. Yes, the technical implementor has in fact a responsibility to consider fairness criteria and ask the question “should we even do this at all?”
What I liked
The book is packed with code examples. I personally learn best when implementing something by hand and seeing how an abstract idea translates to actual source code, so this really matched my learning style.
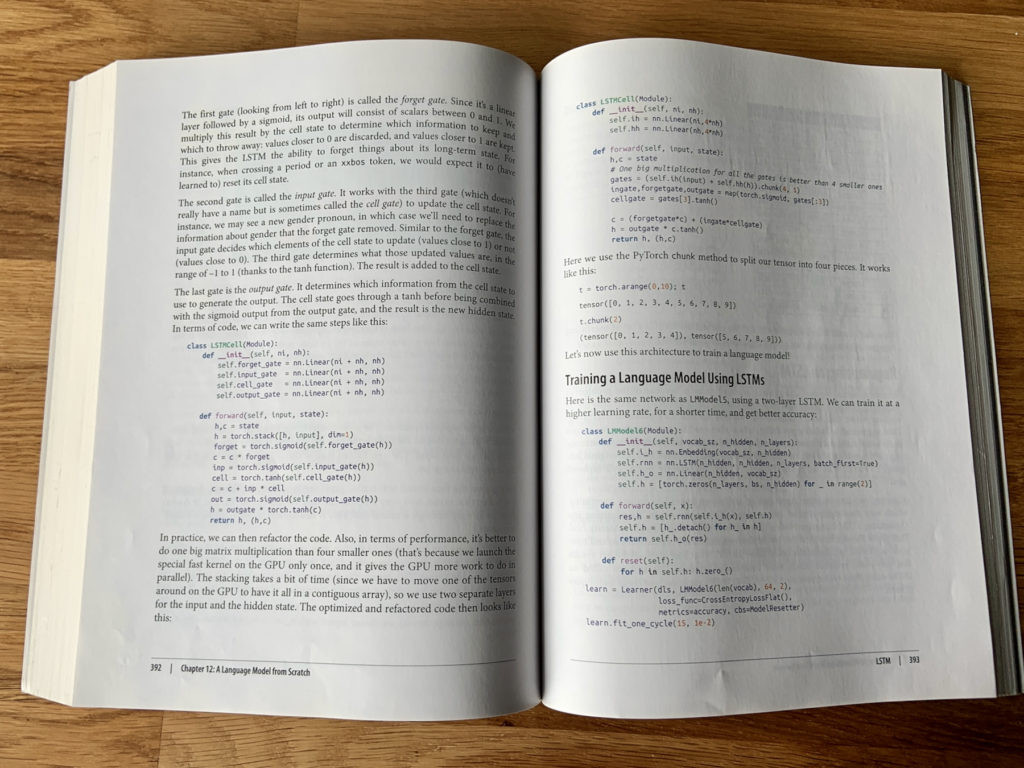
The language of the text is also very easy to digest. You can tell that the fast.ai team wants to teach a little differently and is genuinely excited about the topic. The text is mixed with personal anecdotes and examples of Twitter conversations to create a sense of community around the otherwise technical topic.
The collection of the latest deep learning techniques and condensed experience is immensely valuable: You learn how a proper training process looks like, which techniques you can use to improve the training and how to investigate if the training is not behaving nicely.
What I didn’t like
My biggest gripe with the fast.ai material is their Python coding style: Everything has to be an abbreviation, apparently. I don’t know why you call a parameter ni
when it could just as well be called num_inputs
. If the goal is to “reduce jargon”, using explicit naming in the code would be part of that, if you ask me.
Secondly, the teaching principle of “top-down, then bottom-up” has its quirks: You repeat the same example over and over again, just on different levels of abstraction. When I want to look up “the chapter on convolutional neural networks”, it’s not one chapter I have to browse, but 4 or 5. This may make for a good didactic progression but feels quite repetitive at times.
Who should read the book
The name and subtitle of the book capture it quite well: The code-centric approach of learning (and trying out) deep learning lends itself for people who self-identify as “coders” and not so much as academic scholars who want to have the theory laid out first.
Still, it shows that this book originated in a course. The material will stick if you really follow along and try things for yourself. If you don’t, and you’re completely new to deep learning, it will be hard to map out where in the level of abstractions each chapter is situated.
I actually found the book very helpful for myself, because it helped me understand how to use the latest deep learning technologies such as learning rate finder, 1-cycle training, label smoothing, and mixup augmentation. Having worked with deep learning for a while, I still learned quite some new methods and was able to gain a deeper understanding of concepts I had known before.
Summary
Overall, I really liked the book. The authors did a great job of covering a wide range of deep learning applications while showing both: easy-to-use black box examples and the deepest insides of that black box. This helps to de-mystify the ai hype and teaches helpful hands-on skills.
They share a lot of expert advice on how to set up training procedures properly and I actually agree with their claim: Those who really complete this material have a great starting point working in the field of deep learning.
The didactic style may not be for everyone and I personally hope the fastai coding style doesn’t stick, but I am grateful for the fast.ai team’s contribution: Making deep learning accessible for anyone who is interested.
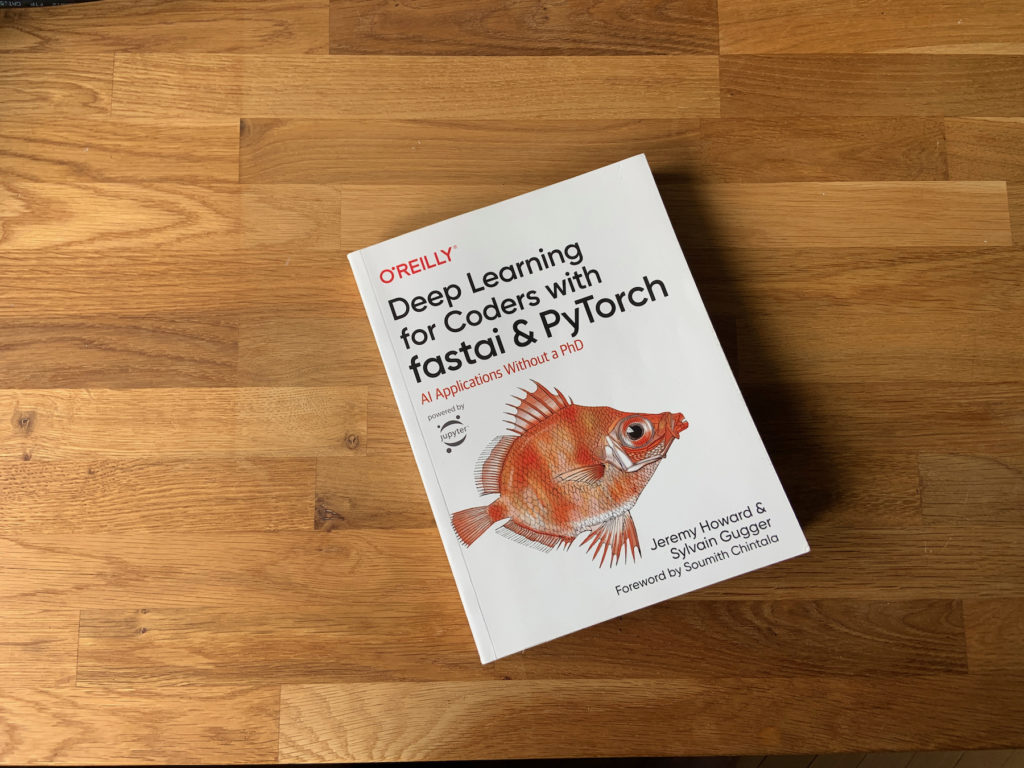